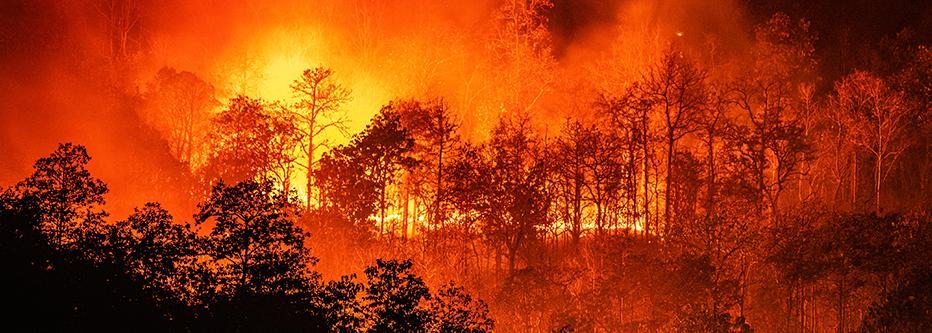
As climate change intensifies, major forest fires are devastating larger areas of the Western U.S. and other arid regions around the world. In addition to threatening lives, properties and ecosystems, these disasters have also contaminated local drinking water supplies as post-fire rain events wash sediment, ash and other toxic materials into surface water bodies.
In order to optimize the performance of water treatment plants under these conditions, Jacobs has successfully applied a machine learning tool – in partnership with the Eugene Water and Electric Board (EWEB) in Oregon – that predicts water quality impacts following forest fires.

Data and machine learning combine to predict the future
Jacobs created the machine learning tool using water quality and operational data collected before and after the Holiday Farm Fire, which began in September 2020 and burned almost 200,000 acres in the Willamette National Forest in Oregon. The fire affected 20% of the McKenzie River watershed, which supplies water to EWEB’s 80MGD Hayden Bridge Water Treatment Plant (WTP) that serves 185,000 people. While EWEB implemented timely actions that minimized water quality impacts, such as erosion control measures and revegetation, increased concentrations of metals, nutrients, solids, bacteria and organic carbon were nevertheless recorded during storm events.
To create the machine learning tool, the team used advanced analytics to identify connections between measured parameters and treatment performance before the fire. These results informed the machine learning model to predict when and how WTP performance would be affected.
Machine learning has proven to be a useful tool in recent years to predict the performance of water treatment plants and optimize operations. Today, most water treatment plants are equipped with sensors that continuously collect data. The process of analyzing collected raw data is difficult and time consuming. However, machines can analyze vast amounts of data and use algorithms to model the complex relations between the various parameters and their effect on the treatment process. Machine learning can be used to create more effective water treatment processes and provide early detection of problems.
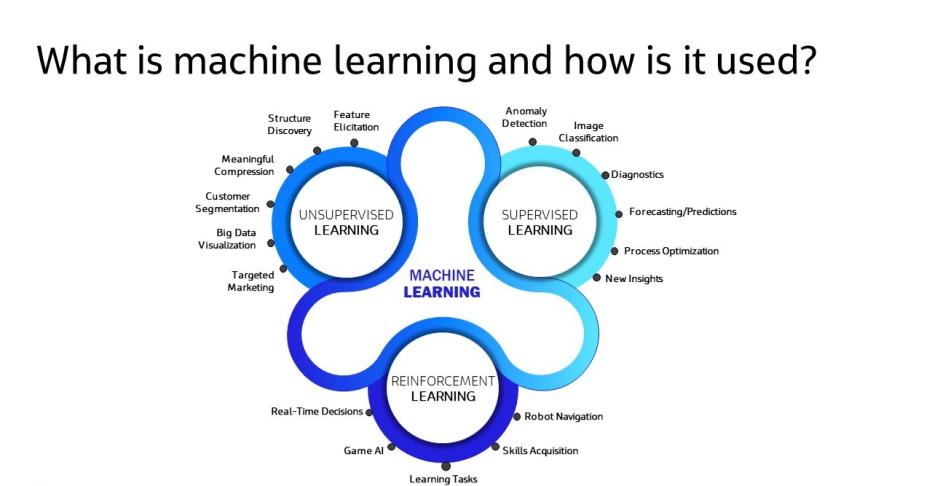
The EWEB model is not the first time that Jacobs successfully applied machine learning tools for water treatment processes. We previously completed a pilot project to optimize chemical coagulation using a predictive neural model for the Woodland Davis WTP in California. Our industry-first Replica digital twin of Singapore PUB’s Changi water reclamation plant also harnesses machine learning capabilities.
Predictive analytics and machine learning are central to our digital OneWater approach at Jacobs. We take a holistic view of the entire water cycle and apply digital tools that optimize client operations and accelerate the digital transformation of the water sector.
The application of machine learning to predict treatment impacts from major forest fires was presented by Kim Ervin, Jacobs Global Technology Leader for Master Planning and Applied Digital Tools during a technical session at the American Water Works Associations’ ACE22 conference in San Antonio, Texas this month. As our industry faces new and complex drinking water quality challenges, the Jacobs team shared insights in a series of technical sessions during the conference – held June 13 – 15th – covering topics such as lead compliance, PFAS and microplastics.
You might be interested in...
-
News
Revisions and Improvements: Navigating the Lead & Copper Rule
The U.S. Environmental Protection Agency’s Lead and Copper Rule Revisions are now effective and the clock has started ticking down to the compliance deadline. At Jacobs, we have a dedicated team of Lead and Copper Rule experts that bring innovative solutions to the challenges imposed by the final rule. Let’s examine the requirements and the actions you need to take.
-
Page
OneWater
Solving the world’s most complex water management challenges requires that we recognize how water connects us and affects us all. It requires different thinking — a OneWater approach — and that’s where we come in.
-
Page
PFAS and Emerging Contaminants
Navigating PFAS and emerging contaminants to enhance health and safety, ensure regulatory compliance and maintain business continuity.
-
News
Jacobs Named 2020 Digital Water Consulting Services Company of the Year by Frost & Sullivan
Digital solutions prove to be more important than ever as water utilities navigate the new normal of COVID-19.
-
Webinars
Identifying Smarter Solutions to Infrastructure Challenges Using Optimization
Infrastructure projects are becoming more challenging as we address aging infrastructure, capacity issues, climate change, population growth and conflicts with other existing infrastructure. Optimization technology employs sophisticated algorithms, enhanced computation and automation to assist water utilities in finding solutions to these complex infrastructure problems that maximize benefits and minimize costs. In this webinar, we will present examples from Anglian Water’s Strategic Pipeline Alliance, a CSO Long-Term Control Plan and others with our partner Optimatics.